Michael Klein
Few issues have seen the hype surrounding artificial intelligence in early 2023. For good reason. Even at this early stage, AI chatbots like GPT4 can impact financial services jobs by automating routine tasks, improving customer support and enhancing professional expertise.
Because chatbots are so-called large language models, they excel at using and analysing language.
Essentially, such models are trained on large amounts of text data from books, articles and websites. They use a neural network to understand grammar, the relationship between words and other language patterns.
At a basic level, large language models attempt to predict the next word in a sentence they generate by using a statistical probability distribution for each potential word and repeat this task over and over again.
The results are no doubt surprising.
Very rarely will a chatbot completely misunderstand a question. The answers provided almost always sound coherent and relevant.
But critics have jumped on the fact that the responses are not at all times accurate.
In fact, chatbots can end up ‘hallucinating’. They can generate answers that sound plausible but are entirely incorrect. This is largely because the answers are based on training data, which includes both accurate and false information, they may lack context or do not cover a time-period relevant to the question or task.
Chatbots are trained to emulate human-like responses and at times prioritise generating creative language over factual accuracy.
While AI solutions will no doubt be held to a higher standard than humans, who make mistakes all the time, it would be a mistake to conclude that current shortcomings would slow the adoption of AI in business processes.
There are several ways these problems can be addressed, both by improving the input and by letting the model self-reflect on the output it provides (see text box).
Text box
Addressing information accuracy in AI chatbots
The “rubbish in – rubbish out” issue involving faulty training data can be overcome by using a more robust dataset.
Chatbots can provide sources to responses, which can then be verified.
Language models can be prompted to use only information verified by multiple trusted sources like scientific studies, news reports from reputable media, encyclopaedias or proprietary data.
In general, companies that are implementing AI chatbots in corporate solutions so far had much success in reducing hallucinations with so-called retrieval augmentation.
This automatically searches and inserts additional databases and documents into the prompts fed to the AI. The process also increases the verifiability of any answers.
In general, chatbots are iterative. They improve based on the feedback they receive, further training and self-learning. This means responses will become more accurate over time.
At this early stage, prompt engineering, the refining of prompts given to the AI. has also led to improved results.
This includes for instance allowing the model to say it does not know the answer, asking for summaries of information rather than answers to specific questions and retrieving opinions included in the data.
ChatGPT and GPT4 are also able to make their reasoning process transparent and take it into account when refining the answer.
This is done by prompting large language models to explain step by step how an answer was derived. Using such prompts and then re-prompting the chatbot to include this in the response improves accuracy immediately.
Prompting the model to produce a variety of answers and then analyse and rate the responses (Tree-of-Thoughts prompting), increases the accuracy even further, to a level on par or even beyond expert human capacity for a wide range of topic areas.
Ultimately, and nowadays that is likely to mean shortly, prompt engineering refinements and fact-checking will no doubt be integrated into AI solutions.
Until then chatbots can be used for supervised baseline research, as well as basic interaction and analysis.
Simple uses of large language models in business
Organisations that want to implement AI chatbots in their business processes are typically concerned about tailoring the AI to their specific use cases and proprietary data, while keeping control of that data and managing the quality of the responses.
At a practical level, preparing proprietary data for machine learning purposes is another challenge.
Overcoming these obstacles will lead to major workflow transformations and higher productivity.
Even using existing public AI chatbots on an individual level can generate productivity gains.
Currently, one strength of large language models lies in analysing large amounts of text data and generating a summary instantly.
For instance, prompting a model to summarise the transcript of an interview, board meeting or any other form of text leads typically to impressive results. Routine tasks, such as recording and transcribing meetings and then producing the minutes, can be taken over almost entirely by AI solutions in a matter of minutes rather than hours.
Chatbots can further supplement research by conducting a risk assessment of individuals, organisations and countries. They can add to that by creating a sentiment analysis on the basis of general or specifically supplied text data.
In addition, AI models can generate text and presentation templates, and analyse tables of data.
Chatbots can output these results to queries in any conceivable format and style and be trained to use a specific tone of voice.
The inclusion of AI solutions in standard personal office software packages (such as Microsoft Copilot) to provide text or data analysis, calendar management and the creation of documents and presentations is only months away.
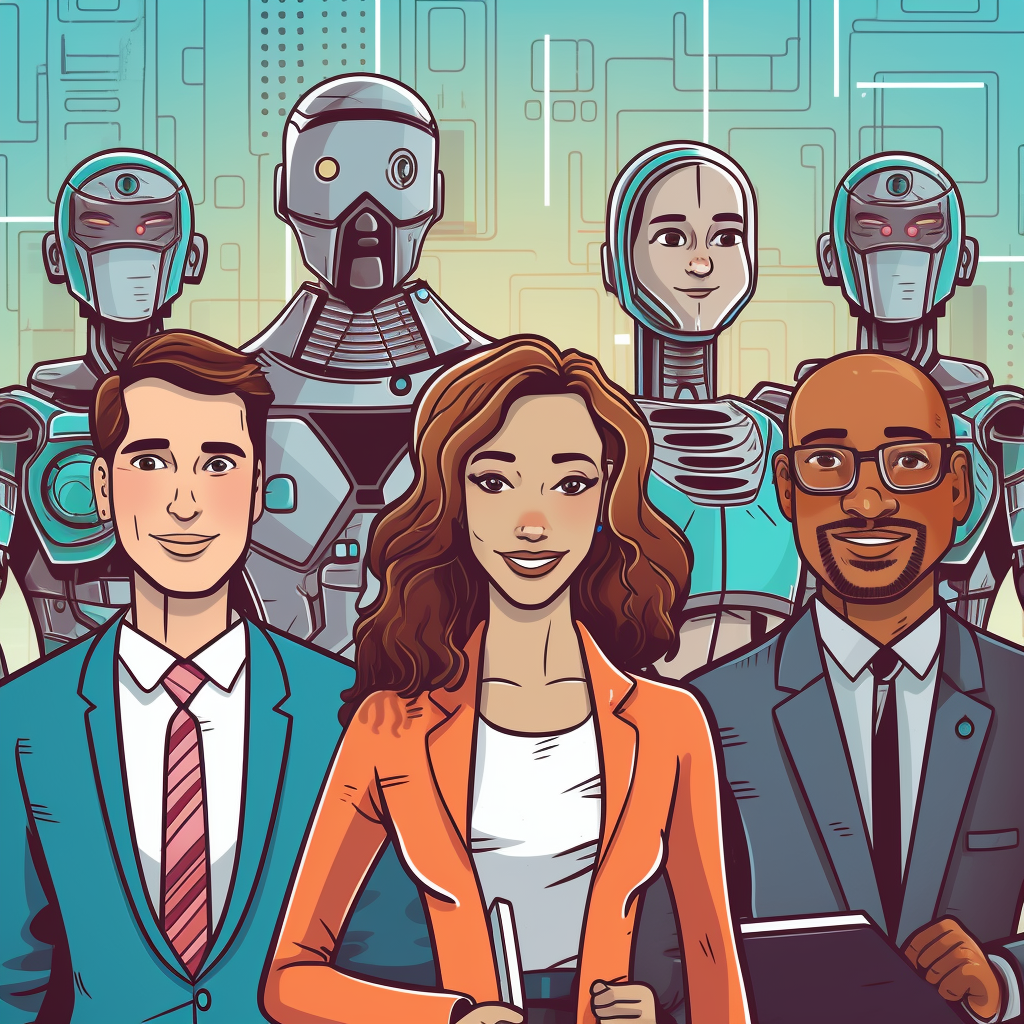
Practical applications in Cayman’s financial services industry
The real business process transformation, however, will result from organisations being able to train AI models for their own specific purposes and uses. Such a generative AI, which is capable of self-learning and executing a number of tasks, is widely expected to boost the efficiency of both individual workers and the companies that employ it.
Traditional AI use cases involve customer onboarding and risk monitoring, including Know Your Customer (KYC) and Anti-Money Laundering (AML) and fraud detection, along with data management.
Banks were first to experiment with chatbots to respond to simple customer queries on their websites and in banking apps. AI-powered chatbots can now provide account balances, transaction histories or process bill payments.
They can be used in marketing to offer financial product information, check customer eligibility, and give basic personal finance advice.
The main areas that are likely to be targeted initially by a wider range of businesses are basic customer interaction, document and data analysis and routine administrative tasks, such as data entry and document processing.
Compliance is another main area that will see administrative tasks taken over by AI solutions. Across financial services firms they can assist with screening for KYC and AML processes.
In client onboarding, AI models can assist by collecting the necessary information and documents, explaining the process, and answering queries. The technology is already used in fraud detection to flag unusual or suspicious transactions and issuing alerts.
For funds they can take care of many investor relations tasks, from providing real-time updates on performance and other key metrics to generating standard reports to investors.
They could also aid with fund subscriptions and redemptions, helping investors through the individual steps and gathering necessary information.
In fund accounting, chatbots can help calculate Net Asset Values (NAVs), manage basic accounting queries, and assist in tracking and categorising transactions.
Some venture capital and private equity funds, in cooperation with accounting firms are using artificial intelligence to pick acquisition targets and start-ups for investment.
These solutions scrutinise financial statements, sell-side research, earnings transcripts and pitch decks and produce the results in concise briefings.
In legal services chatbots can provide rapid, accurate responses to basic client inquiries, answering general legal questions, offering case updates, or guiding clients through legal processes. They can generate documents or analyse contracts for key clauses and legal risks.
The fact that law firms collect legal documents in knowledge banks plays into how an AI analyses and repurposes text and can be used for preparing standard contracts. In addition, they can be employed to analyse judgments and orders to predict the outcome of pending cases.
These are just some of the examples, where the use of AI models is currently contemplated or implemented. The speed at which large language models have improved just over the last six to nine months has taken many by surprise. It shows that many more novel use cases are likely to emerge.
Substitute and complement
The consensus is that, at this stage, AI solutions will mainly serve as assistants to financial services professionals. In other words, just another tool that will free up time for more complex tasks.
A much-quoted research note by Goldman Sachs published in March predicts up to 300 million jobs worldwide could be displaced by AI.
It still comes to the conclusion that generative AI will “sometimes substitute but often complement existing work”.
However, the indications are that repetitive, simple, document-heavy, administrative tasks are under threat to be replaced by automated processes in the future. In the US, Goldman Sachs estimated one fourth of all work tasks could be eliminated with administrative (46%) and legal professions (44%) being the most impacted.
The analysis found that business and financial operations have an above average exposure to automation and more than a third of positions could be eliminated (35%).
Of course, it is not clear whether these estimates are even close to correct or how long such a displacement would take. The Economist recently noted that historically the projected job destruction caused by new technology happened far slower than initially thought, because of obstacles to technology adoption in the workplace ranging from regulation to worker resistance.
Goldman Sachs pointed out that, in the past, worker displacement has been offset by the creation of new jobs and added that overall the impact of AI could lead to a productivity boom.
So far technology has impacted labour in contradictory ways. On the one hand it resulted in the substitution or displacement of tasks, functions and roles and on the other it caused productivity gains that reduced the costs of goods and services, which raised incomes and generated demand in other sectors of the economy.
On an individual level, however, the displacement effect of technology comes at a cost that has the potential to cause social problems before any efficiency gains have filtered through the economy and produced new opportunities.
Sources:
Stephen Wolfram, What is ChatGPT doing and why does it work?, 14 Feb. 2023
Tree of Thoughts: Deliberate Problem Solving with Large Language Models, Shunyu Yao, Dian Yu, Jeffrey Zhao, Izhak Shafran, Thomas L. Griffiths, Yuan Cao, Karthik Narasimhan, 17 May 2023
Goldman Sachs, The Potentially Large Effects of Artificial Intelligence on Economic Growth (Briggs/Kodnani), 26 March 2023
The Economist, Your job is (probably) safe from artificial intelligence, 7 May 2023